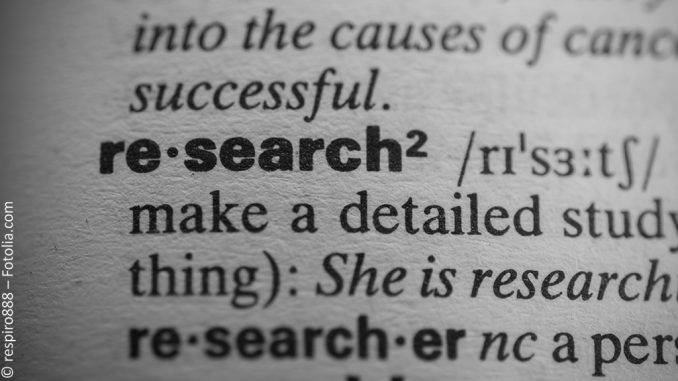
Trustworthiness is the highest premise of good research. But when is a paper considered trustworthy? Quantitative and qualitative research have different criteria for trustworthiness – we explain them to you.
„A man is more likely to believe something if he would like it to be true.“
Francis Bacon
Quantitative and qualitative research is based on different research paradigms that reflect the researcher’s scientific worldview. Due to this, methodology, methods and results naturally differ accordingly (Goertz & Mahoney, 2012). In order to appreciate the quality of academic research, it makes sense to apply different criteria for (1) quantitative and (2) qualitative methods.
1. Trustworthiness of quantitative research
Bryman and Bell (2005, p. 154) define quantitative research as „entailing the collection of numerical data and exhibiting the view of relationship between theory and research as deductive, a predilection for natural science approach, and as having an objectivist conception of social reality“. Data is usually generated by means of surveys and experiments and analyzed through statistical tests such as ANOVA (e.g. Black, 1999) and Cohen’s Kappa (Cohen, 1980).
Therefore, its quality can be assessed by its (a) internal and (b) external validity, (c) reliability and (d) objectivity (Lincoln & Guba, 1985), which are described below:
a. Internal validity
A study is internally valid if it is able to determine whether a causal relationship exists between one or more independent variables and one or more dependent variables (Heffner, 2017), i.e. it is explanative. A study is internally valid if there are as little confounding variables as possible. Confounding variables are variables that the researcher fails to control or eliminate, allowing the results to show false correlation (Shuttleworth, 2008).
Therefore, on the one hand, internal validity refers to how well the study is run, e.g. research design, operational definitions, how variables are measured, and what is (not) measured (Huitt, Hummel & Kaeck, 1999). On the other hand, internal validity determines how confidently it can be concluded that the change in the dependent variable was produced solely by the independent variable as opposed to extraneous ones (ibid.).
Campbell and Stanley‘s (1966) seminal work on experimental and quasi-experimental designs identifies and describes eight threats to internal validity:
- History – Studies that collect data over long time periods are likely to be affected by research subjects’ unique experiences over time that function like extra and unplanned, therefore independent variables.
- Maturation – Like the above, this effect also draws on the normal passage of time. Research subjects may become more or less motivated and thus affecting the internal validity of the study.
- Testing – Research design often use pre-tests. These may change, i.e. contaminate the outcome of the actual study.
- Instrumentation – Changing measurement methods or their administration may affect what is measured.
- Statistical Regression – Re-testing research subjects that were originally chosen because of extremely high or low scores can be expected to produce a distribution closer to the entire population’s distribution.
- Selection – The results of the study will be biased if the research subjects in the control and experimental groups are different from another at the beginning of the study.
- Experimental Mortality – If the comparison groups experience different or high levels of withdrawal (mortality) of research subjects, it becomes questionable whether the observed differences between the groups are due to different or high drop-out rates or are produced by the independent variable.
- Selection Interactions – If the selection method of research subjects interacts with one or more of the above threats, the study’s results will be biased.
b. External validity
External validity describes the ability to generalize a study, which is particularly threatened if people, places, or times are poorly chosen (Trochim, 2006).
As measuring an entire population is impossible, a sample, or a subset of the population is studied. The sample chosen needs to represent the whole population in order to allow inferences to be drawn (Landreneau, 2009).
A good sampling model firstly identifies the population that it should generalize, subsequently drawing a sample from that population, conducting research and finally generalizing the results back to the original population (Trochim, 2006). External validity will improve the more a study is replicated (ibid.).
The sufficient sample size depends on the minimum number of participants required to identify a statistically significant difference and increases the smaller the anticipated effect is (Burmeister & Aitken, 2012).
As quantitative methods are often used in natural science, the very fine tools and criteria developed for that realm may be adapted and used for business research.
As an example, a set of eight criteria was developed to identify high quality evidence in the public health sector (Effective Public Health Practice Project, 1998). Each of the criteria (selection bias, study design, confounders, blinding, data collection methods, withdrawals and dropouts, intervention integrity, analysis) is rated as strong, moderate, or weak, thus achieving an overall methodological rating (ibid.).
c. Reliability
In research, reliability means “repeatability” or “consistency”; it is achieved if a measure will always provide the same result (Trochim, 2006). Reliability issues often come up when researchers adopt a subjective approach to research (Wilson, 2010), which on the other hand is consciously allowed in qualitative research.
d. Objectivity
Objectivity demands that „researchers should remain distanced from what they study so findings depend on the nature of what was studied rather than on the personality, beliefs, and values of the researcher“ (Payne & Payne, 2004).
The four criteria for trustworthiness of quantitative research overlap at some points, for example validity and reliability: if a study’s results can be generalized, its repetition should offer the same results.
2. Trustworthiness of qualitative research
The above described criteria applying for quantitative research are not suitable for qualitative research as it accepts multiple and subjective realities and aims at deep insights. Therefore, these criteria are not sought to be complied with. In order to provide a different set for criteria that can be used for ascertaining the quality, Lincoln & Guba (1985) created a corresponding set of criteria for trustworthiness of qualitative research: (a) credibility (vs. internal validity), (b) transferability (vs. external validity), (c) dependability (vs. reliability) and (d) confirmability (vs. objectivity).
a. Credibility
Credibility depends on the richness of the data and analysis and can be enhanced by triangulation (Patton, 2002), rather than relying on sample size aiming at representing a population.
There are four types of triangulation as introduced by Denzin (1970), which can also be used in conjunction with each other:
- Data triangulation – using different sources of data, e.g. from existing research
- Methodological triangulation – using more than one method, e.g. mixed methods approach, however with focus on qualitative methods
- Investigator triangulation – using more than one researcher adds to the credibility of a study in order to mitigate the researcher’s influence
- Theoretical triangulation – using more than one theory as conceptual framework
b. Transferability
Transferability corresponds to external validity, i.e. generalizing a study’s results. Transferability can be achieved by thorough description of the research context and underlying assumptions (Trochim, 2006). With providing that information, the research results may be transferred from the original research situation to a similar situation.
c. Dependability
Dependability aims to replace reliability, which requires that when replicating experiments, the same results should be achieved. As this would not be expected to happen in a qualitative setting, alternative criteria are general understandability, flow of arguments, and logic. Both the process and the product of the research need to be consistent (Lincoln & Guba, 1985).
d. Confirmability
Instead of general objectivity in quantitative research, the researcher’s neutrality of research interpretations is required. This can be achieved by means of a confirmability audit that includes an audit trail of raw data, analysis notes, reconstruction, and synthesis products, process notes, personal notes, as well as preliminary developmental information (Lincoln & Guba, 1985).
The approach to sampling differs significantly in quantitative and qualitative research. Qualitative samples are usually small and should be selected purposefully in order to select information-rich cases for in-depth study (Patton, 2002). There may be as few as five (Creswell, 1998, p. 64) or six participants (Morse, 1994, p. 225).
As seen from the above criteria, qualitative research requires far more documentation than quantitative research in order to establish trustworthiness in. Quantitative research, on the other hand, requires more effort during the research design phase.
With qualitative and quantitative research serving different objectives and being designed in a different way, quality assessment criteria must be adapted and adhered to accordingly.
Literature:
- Bacon, F. (1620). The New Organon: or True Directions Concerning the Interpretation of Nature. Retrieved from: http://www.constitution.org/bacon/nov_org.htm
- Black, T. (1999). Doing Quantitative Research in the Social Sciences – An Integrated Approach to Research Design, Measurement and Statistics. London, Sage.
- Burmeister, E. & Aitken, L. M. (2012). Sample size: How many is enough? Australian Critical Care, 25(4), pp. 271-274. doi: 10.1016/j.aucc.2012.07.002
- Campbell, D. T., & Stanley, J. C. (1966). Experimental and quasi-experimental designs for research. Chicago: Rand McNally.
- Creswell, J. W. (1998). Qualitative inquiry and research design: Choosing among five traditions. Thousand Oaks, CA: Sage.
- Cohen, J.: Weighted kappa: Nominal scale agreement with provision for scaled disagreement or partial credit. In: Psychological Bulletin. 1968, 213–220.
- Goertz, G. & Mahoney, J. (2012). A Tale of Two Cultures: Qualitative and Quantitative Research in the Social Sciences. Princeton, NJ: Princeton University Press.
- Lincoln, Y. S. & Guba, E. G. (1985). Naturalistic inquiry. Beverly Hills, CA: Sage.
- Effective Public Health Practice Project (1998). Quality Assessment Tool For Quantitative Studies. Hamilton, O.N.: Effective Public Health Practice Project. Available from: http://www.ephpp.ca/index.html
- Heffner, C. (2017): Research Methods. Retrieved from: https://allpsych.com/researchmethods/experimentalvalidity/
- Huitt, W., Hummel, J. & Kaeck, D. (1999). Internal and External Validity. Retrieved from: http://www.edpsycinteractive.org/topics/intro/valdgn.html
- Landreneau, K. J. (2009). Sampling Strategies. Retrieved from: http://www.natco1.org/research/files/samplingstrategies.pdf
- Morse, J. M. (1994). Designing funded qualitative research. In N. K. Denzin & Y. S. Lincoln, Handbook of qualitative research (pp. 220-235). Thousand Oaks, CA: Sage.
- Payne, G. & Payne, J. (2004). Key Concepts in Social Research. SAGE Key Concepts.
- Patton, M. Q. (2002). Qualitative evaluation and Research Methods 3rd ed. Newbury Park: Sage.
- Shuttleworth, M. (2008): Research Methodology. Retrieved from: https://explorable.com/confounding-variables
- Trochim, W. M. (2006). Social Research Methods. Retrieved from Research Methods Knowledge Base: https://www.socialresearchmethods.net/
- Wilson, J. (2010). Essentials of Business Research: A Guide to Doing Your Research Project. London: Sage.
The author is responsible for the content and form of this article.