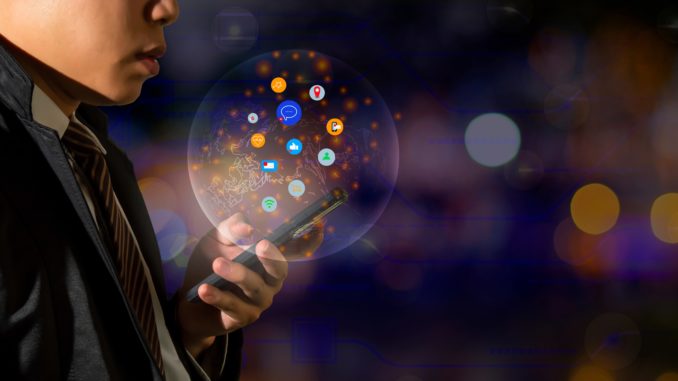
Due to the flood of information and the resulting inability of consumers to make decisions, recommender systems are increasingly becoming a key tool in e-commerce. But how do these systems influence consumers’ purchase intentions? A new study conducted as part of a Master’s thesis at Munich Business School examines this question using the example of Taiwan.
“Being digitally connected substantially changes the way companies compete and succeed.”
Jung, Kraft, 2017
With advancements in information technology, e-commerce plays an important role in our daily lives and provides consumers with a great deal of convenience. However, consumers tend to become overwhelmed by the ever-growing amount of product-related content they receive, and suffer from information overload, which may lead to poor decision making. To cope with this information overload, recommender systems are increasingly becoming a key tool in e-commerce. The main feature of recommender systems is their ability to analyze user behavior, especially which item a particular user might be interested in, and further provide users with item suggestions according to their preferences and behaviors (Lu, Wu, Mao, Wang, & Zhang, 2015, p. 12). To generate recommendations, recommender systems follow the process that consists of three phases. In the first phase, the system collects extensive user information to gain a deeper understanding of the target users and lay a solid foundation for future phases. This input can be either explicit feedback, such as product ratings, or implicit feedback, such as purchase history. In the second phase, the recommender system filters and utilizes the user data collected in the first phase by adopting a machine learning algorithm. Lastly, the system predicts and presents item recommendations to the users (Isinkaye, Folajimi, & Ojokoh, 2015, pp. 263-264).
Recommender systems – a powerful tool for decision making and influencing purchase intention
Based on the data filtering and rating estimation methods, recommender systems are usually divided into the following categories: content-based, collaborative and hybrid filtering systems. In the content-based filtering technique, recommendation is generated by matching up the contents of items and the users’ profiles. The content of items is represented as a set of descriptions which areextracted from the features of items. The user profile consists of the information about the interests, preferences and needs of the user and is constructed by analyzing the features of items that the user has purchased or viewed in the past (Lops, Gemmis, & Semeraro, 2011, p. 75). Collaborative filtering considers the opinions of other users as important factor and proposes recommendations to a user based on the analysis of other users who have similar preferences (Sivapalan, Sadeghian, Rahnama, & Madni, 2014, p. 163). This approach requires historical data and past ratings of users and works by building a database made up of users’ preferences for items and searching the users who have more in common with the target user (Cheng, Wang, 2014, p. 290). Hybrid filtering was proposed by combining two or more recommendation techniques to achieve higher performance and to avoid the limitations of the individual techniques it combines (Isinkaye, Folajimi, & Ojokoh, 2015, p. 269). Most commonly, the hybrid filtering approach combines the strengths of the content-based and collaborative filtering approaches to optimize recommender systems (Bagherifard, Rahmani, Nilashi, & Rafe, 2017, p. 1777).
This study – conducted as part of a final thesis at Munich Business School – sets out to examine the factors related that affect consumers’ purchase intention toward the products suggested by recommender systems in online shopping and gain a holistic view of recommender systems from the consumers’ perspective. To this end, our research model integrates the unified theory of acceptance and use of technology (UTAUT) model proposed by Venkatesh, Morris, Davis, & Davis (2003) with the internet users’ information privacy concerns (IUIPC) model proposed by Malhotra, Kim, & Agarwal (2004). The UTAUT model helps to understand users’ perceptions and behavioral intentions towards recommender systems, while the IUIPC model explores users’ privacy concerns regarding recommender systems. Furthermore, to better understand what makes a recommendation useful to consumers, this research model includes the three factors that influence performance expectancy, i.e., recommendation accuracy, novelty, and diversity.
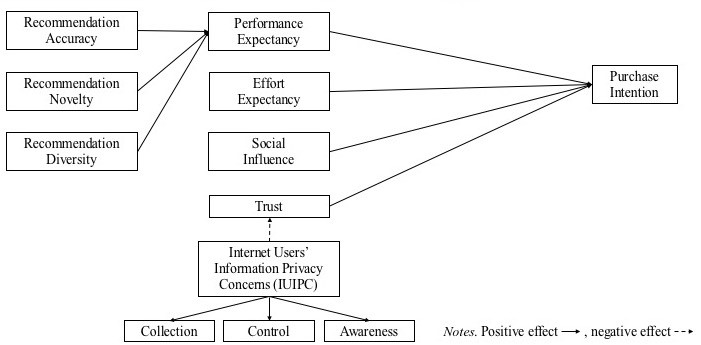
For the purpose of investigating the determinants of Taiwanese online shoppers’ intention to purchase recommended products, the quantitative approach was chosen to develop the survey. The online survey was distributed via social media platforms and messaging applications, and a total of 276 complete and valid responses were collected.
How effective recommender systems should be designed and developed
The findings indicate that consumers’ trust in recommender systems and performance expectancy of recommender systems are important factors influencing consumers’ purchase intention and that recommendation accuracy, novelty, and diversity indirectly affect purchase intention by shaping consumers’ perceptions about the performance expectancy of recommender systems. Thus, this study makes several suggestions for practices to facilitate the design and development of effective recommender systems by service providers.
Building consumer trust
- Ensure that the proposed recommendations prioritize the interests of the consumer over those of the company. Since recommender system narrows the options for consumers, it may, to some extent, nudge consumers in the desired direction of the service provider.
- Handle product reviews with caution and weed out fake reviews and ratings in order to provide products with credibility and authenticity. Given that product ratings are important input for recommender systems to generate recommendations, if the service providers are not aware of the problem of fake reviews and address it, the products suggested by the recommender systems are likely to have fraudulent reviews, resulting in a loss of consumers’ trust.
Improving the usefulness of recommender systems
- Enhance the ability of recommender systems to immediately identify consumers’ current interests and needs in order to support consumers in finding the ideal products quickly and help them increase the efficiency of product search and decision making.
- Optimize the machine learning models for more desirable recommendations, and strike a balance between recommendation accuracy, novelty, and diversity, rather than solely focusing on one of them.
This study compares Generation X and Generation Y consumers in Taiwan, as the online shopping penetration rates for both generations are higher than for any other age group and have the potential to continue growing. It is worth noting that consumers belonging to different generational cohorts have different perceptions toward recommended products, resulting in different standards for the usefulness of recommender systems. As a result, service providers should distinguish the generational cohort to which consumers belong before proposing recommendations, so as to present recommended products according to the characteristics of each generational cohort and effectively enhance consumers’ purchase intention. To give consumers peace of mind with a secure shopping experience, service providers should also enable consumers to have control over personal data and recommendations, clearly disclose their privacy policies, and keep consumers informed about the way in which recommender systems collect and use data.
With this blog post we want to motivate students to further analyze new features and functionalities of digital customer experience touchpoints such as websites, digital platforms, apps, chat bots and more. First of all, this is absolutely relevant, but in many areas still uncharted area for researchers. Secondly, students will benefit for understanding customer acceptance of new digital touchpoints and gain deep insight which will be a real booster for their further research or business career.
Sources:
- Bagherifard, K., Rahmani, M., Nilashi, M., & Rafe, V. (2017). Performance improvement for recommender systems using ontology. Telematics and Informatics, 34(8), pp. 1772-1792.
- Cheng, L., Wang, H. (2014). A fuzzy recommender system based on the integration of subjective preferences and objective information. Applied Soft Computing, 18, pp. 290-301.
- Isinkaye, F., Folajimi, Y., & Ojokoh, B. (2015). Recommendation systems: Principles, methods and evaluation. Egyptian Informatics Journal, 16(3), pp. 261-273.
- Jung, H.H., Kraft, P. (2017). Vorwort, in, Jung, H.H., Kraft, P.: Digital vernetzt. Transformation der Wertschöpfung. Szenarien, Optionen und Erfolgsmodelle für Geschäftsmodelle, Produkte und Services, pp. 5-11. Munich, Hanser.
- Lops, P., Gemmis, M. D., & Semeraro, G. (2011). Content-based Recommender Systems: State of the Art and Trends. In Ricci F., Rokach L., Shapira B., Kantor P. (Eds.), Recommender Systems Handbook (pp. 73-105). Boston, MA: Springer.
- Lu, J., Wu, D., Mao, M., Wang, W., & Zhang, G. (2015). Recommender system application developments: A survey. Decision Support Systems, 74, pp. 12-32.
- Malhotra, N. K., Kim, S. S., & Agarwal, J. (2004). Internet Users’ Information Privacy Concerns (IUIPC): The Construct, the Scale, and a Causal Model. Information Systems Research, 15(4), pp. 336-355.
- Sivapalan, S., Sadeghian, A., Rahnama, H., & Madni, A. M. (2014). Recommender systems in e-commerce. 2014 World Automation Congress (WAC), pp. 179-184.
- Venkatesh, V., Morris, M. G., Davis, G. B., & Davis, F. D. (2003). User Acceptance of Information Technology: Toward a Unified View. MIS Quarterly, 27(3), pp. 425-478.
The authors are responsible for the content and form of this article.

You are interested in economics and want to acquire in-depth business know-how?
Then the international business degrees at Munich Business School (MBS) are just right for you! At MBS you won’t cram dry theory from old textbooks, but learn in a outcome-oriented way and gain valuable practical experience. Convince yourself:
Bachelor’s in International Business
Master’s in International Business
Master’s in International Business I Finance
Master’s in Innovation and Entrepreneurship
Master’s in International Marketing and Brand Management
Master’s in Sports Business and Communication
MBA General Management
Doctor of Business Administration